
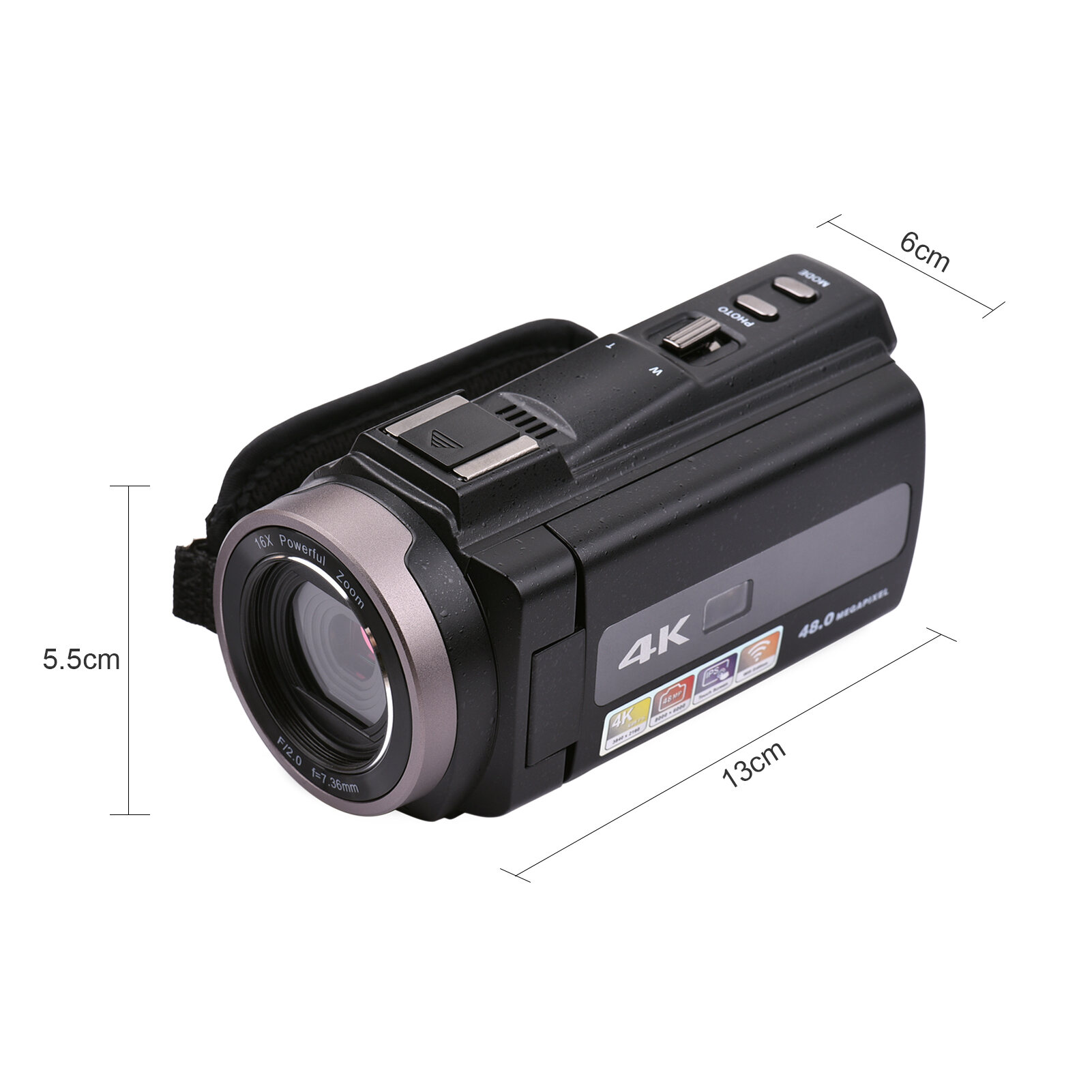
In International Conference on Machine Learning 524–533 (PMLR, 2019). Noise2Self: blind denoising by self-supervision. In International Conference on Machine Learning 2965–2974 (PMLR, 2018).īatson, J. Noise2Noise: Learning image restoration without clean data. Applications, promises, and pitfalls of deep learning for fluorescence image reconstruction. Content-aware image restoration: pushing the limits of fluorescence microscopy. Reducing the dimensionality of data with neural networks. We trained our denoiser on all ‘ses-perceptionTraining’ sessions across five individuals (three perception training sessions, ten runs each). To train the fMRI denoiser, we used datasets that can be downloaded from an S3 bucket: arn:aws:s3:::/ds001246. Before applying DeepInterpolation, we extracted 3,600 s of data from each of the recordings listed in Supplementary Table 1.
#Video 5 cm full movie Offline#
Median subtraction was applied to the DAT files after processing, but the DAT files do not include an offline high-pass filter. Neuropixels raw data can be accessed by experiment ID and probe ID using the following paths: visual-coding-neuropixels/raw-data///spike_band.dat. Some experimental data have not been released to S3 at the time of publication. The majority of these experiment IDs are available in the S3 bucket.

The list of used experiment IDs is available in JSON files (in deepinterpolation/examples/json_data/) on the DeepInterpolation GitHub repository. We used a random subset of 1,144 experiments for training the denoising network for Ai93, and 397 experiments were used for training the denoising network for Ai148. Two-photon imaging files can be accessed according to experiment ID using the following path: visual-coding-2p/ophys_movies/ophys_experiment_>.h5. Two-photon imaging and Neuropixels raw data can be downloaded from the following S3 bucket: arn:aws:s3:::allen-brain-observatory. We anticipate that DeepInterpolation will provide similar benefits in other domains in which independent noise contaminates spatiotemporally structured datasets. Denoising was attained without sacrificing spatial or temporal resolution and without access to ground truth training data.

Extracellular electrophysiology recordings processed with DeepInterpolation yielded 25% more high-quality spiking units than those computed from raw data, while DeepInterpolation produced a 1.6-fold increase in the SNR of individual voxels in fMRI datasets. Applying DeepInterpolation to two-photon calcium imaging data yielded up to six times more neuronal segments than those computed from raw data with a 15-fold increase in the single-pixel signal-to-noise ratio (SNR), uncovering single-trial network dynamics that were previously obscured by noise. Here, we introduce DeepInterpolation, a general-purpose denoising algorithm that trains a spatiotemporal nonlinear interpolation model using only raw noisy samples. Technologies for measuring neural activity (calcium imaging, extracellular electrophysiology and functional magnetic resonance imaging (fMRI)) operate in domains in which independent noise (shot noise and/or thermal noise) can overwhelm physiological signals. Progress in many scientific disciplines is hindered by the presence of independent noise.
